EuroDEM 2018 Abstracts
Access oral, workshop and seminar, and poster abstracts from EuroDEM 2018.
Oral Abstracts
Wolfgang Gaissmaier
An Adaptive Toolbox for Diagnostic Decision Making: Smart Heuristics, Social Intelligence and Transparent Representations
Diagnosticians face many aspects of risk and uncertainty when making decisions. They need to understand the benefits and harms of employing diagnostic tests, for instance for the early detection of disease, and they need to be able to understand the meaning of test results. Furthermore, they need to identify patients at risks quickly and, more generally, find the right diagnosis for each patient. None of these tasks is trivial, and the literature has identified various pitfalls and systematic errors. But the decision sciences have also developed methods to support diagnosticians. Importantly, research has shown that there is not one tool to solve all diagnostic tasks, but that diagnostic decision making needs to be an adaptive process where the right decision making tool is applied to the right task. The adaptive toolbox for successful diagnosticians includes transparent representations for understanding statistical information, smart heuristics for dealing with uncertainty, and social intelligence, which is particularly useful when pooling several independent judgments.
Gerd Gigerenzer
Medical Decisions and Heuristic Reasoning
Diagnostic errors contribute substantially to patient morbidity and mortality. Among the many factors contributing to diagnostic error, cognitive errors of the diagnosing physician have received considerable attention. Many authors have attributed cognitive errors to the use of fast, intuitive thinking and consequentially advocated the implementation of strategies that enforce deliberate considerations. However, the notion of a general superiority of slow analytic thinking in medical decision making has been contested recently. For example, a number of studies correlating diagnostic accuracy with time to diagnosis have found strong inverse relationships, where faster diagnoses were associated with higher quality of diagnoses. Additionally, human “intuition” has evolved well over time and developed a number of mechanisms that work quite well in uncertain and probabilistic environments. A particularly successful approach of strategies neglecting the vast majority of potentially available information and instead focusing on few key characteristics are so called heuristics. After a lifetime of research devoted to heuristic reasoning and information literacy, Gerd Gigerenzer is in a unique position to familiarize the audience with the “fast and frugal” heuristic approach in general and in diagnostic reasoning specifically and contrast his views with extensive research into information literacy of physicians, arguably an important prerequisite for any more “analytic” approach.
Olga Kostopoulou
Diagnostic Decision Support in General Practice
Missing a serious diagnosis, such as a cancer, is the most important kind of diagnostic error in general practice because it can have the worst consequences for patients: inappropriate or delayed treatment can directly affect survival. I will describe the research that underlay the design of a diagnostic support system for General Practitioners (currently in prototype form), and how it was evaluated in different studies in both low and high-fidelity simulations. I will also discuss GPs’ and patients’ attitudes to diagnostic support and possible ways to improve acceptance of external aids by decision makers.
Christina Naaktgeboren
To err on the side of uncertainty
The terms overdiagnosis, underdiagnosis, and diagnostic error are increasingly used in many different clinical contexts, with varying definitions and different contributing mechanisms, leading to troublesome communication. A conceptual framework is presented describing the overarching problems surrounding tests into different types of uncertainty and errors. Briefly, uncertainty about whether more good is being done than harm may exist at several critical decision points: who should receive a test, how to precisely define a disease and its subtypes, and how should a condition be managed? Alongside these uncertainties, various errors can occur. This framework assists researchers to think more carefully about the multiple underlying key mechanisms leading to problems jointly labeled as overdiagnosis or underdiagnosis and diagnostic error.
Kerstin Ritter
Machine learning in radiology
Machine learning techniques, including deep learning, seem to have a great potential in reducing diagnostic errors in radiology, After an introduction into different causes of diagnostic errors and key ideas of machine and deep learning, this talk will show several applications in the medical imaging field with a focus on neuroimaging, including own work on multiple sclerosis and different types of dementia. Challenges in using machine learning such as the need for much data and the black box-problem will be discussed.
Sue Sheridan
Diagnostic Error from a Patient Perspective
The presentation, “Diagnostic error from a patient perspective” offers an overview of 2 cases studies of diagnostic error through the lens of a mother and widow who has partnered with the healthcare system to prevent harm from missed, wrong and delayed diagnosis. The presentation will map the diagnostic journeys of her son, Cal, and late husband, Pat, onto the National Academy of Medicine’s Diagnostic Process diagram to illustrate the complexities of diagnosis as well as the resultant “what ifs” that patients, clinicians and healthcare systems ask themselves after a diagnostic error that can serve as catalysts for diagnostic improvement efforts.
The presentation will also offer an example of the power of patients partnering with researchers, educators, policy makers, NGOs, public health officials, quality assurance organizations and healthcare systems that resulted in a national change in the standard of care of newborn jaundice management in the USA.
As the Director of Patient Engagement for the Society to Improve Diagnosis in Medicine (SIDM), Sue Sheridan will also share how she is leveraging patient perspectives to improve all facets of SIDM’s diagnostic improvement efforts.
Hardeep Singh
Safer Systems For Safer Diagnosis: Lessons From a Decade of Research to Help Pave the Road Ahead
This presentation will discuss challenges and opportunities related to understanding and reducing diagnostic error through health system-centric approaches (i.e. focusing more than just what’s in “the doctors head” on broader system-related factors that inevitably influence these thought processes). Topics will include the use of health information technology and data from electronic health records, organizational approaches to measure the problem of diagnostic error, and application of learning health systems approaches to understand and improve diagnosis.
Workshop 1
Marie-Claude Audetat/Mathieu Nendaz
Diagnosing and Remediating Diagnostic Error in Residency Education
Between 5% and 15% of medical students and residents suffer from academic difficulties; unfortunately, these difficulties are often recognized late in the learners’ course of study and training, usually when problems arise in clinical rotations.
Studies on diagnostic errors and difficulties, at undergraduate and postgraduate levels, indicate that a majority of errors include a cognitive component.
According to Graber et al. (2005), the majority of cognitive difficulties are not directly related to a lack of knowledge, but rather to a flaw in data collection, data integration, and data verification.
Limiting a remediation process to the sole knowledge dimension may thus prove insufficient to address reasoning difficulties. Many studies indicate that delayed or poor identification and remediation of clinical reasoning difficulties can lead to clinician underperformance and can ultimately, risk compromising patient care.
Identifying and delineating concerns early may facilitate timely remediation of problems. Nevertheless, it may be difficult for teachers to take on this educational role. Another challenge for supervisors is the fact that they only have access to the result of learners’ clinical reasoning process (e.g. the diagnosis or management plan) or to the manifestations of various steps of the process (e.g. how a learner takes a history based on diagnostic hypotheses). Clinical supervisors must therefore possess sufficient understanding of clinical reasoning and strong pedagogical skills to be able to interpret these manifestations and recognize cues of reasoning difficulties. This will enable them not only to rapidly detect and diagnose potential reasoning difficulties but also to articulate their findings to their learners.
This workshop will focus on supervising skills aiming at recognizing and remediating reasoning difficulties which may lead to diagnostic errors in trainees. A taxonomy of the most common clinical reasoning difficulties, (as they present in learners’ patient encounters, case summaries, or chart review) will be presented in this workshop: we will describe the educational process of supporting clinical reasoning and diagnosing clinical reasoning difficulties in the clinical setting. We will then focus on the management of these difficulties, providing a more detailed description and specific remediation strategies. Participants will be invited to work on how to develop a pedagogical diagnosis and focused remediation strategies. Concrete examples of supervision will be proposed and discussed.
Workshop 2
Jason Maude, Gordon Caldwell, Tobias Mueller
Practical Solutions to Diagnostic Error
Implementing solutions, effecting change
This session aims to provide participants with practical ideas and advice about how to improve diagnostic decision making. The panel includes seasoned practitioners from primary, secondary and tertiary care who will talk about the particular issues they face and solutions they use and have tried.
They will also talk about the all-important cultural issues which often hinder diagnostic improvement initiatives within an institution and how they have tackled them. There will be plenty of time left for questions and discussion.
Learning objectives:
- Describe possible solutions to improve diagnosis across primary,
secondary and tertiary care - Understand the issues involved with implementing strategies and tools to improve diagnosis
Tobias Mueller
Diagnostic decission Support in Undiagnosed and Rare Diseases
Dr. Tobais Mueller will give a case based presentation on how computerized diagnostic decission support tools can assist in undiagnosed and rare diseases. State of the art tools will be presented, chances highlighted and limitations discussed.
Seminar 3
Paul Epner, Mario Plebani, Cécile Ravesloot
When diagnostic testing leads to harm
In this session, diagnostic professionals will talk about how errors in the laboratory testing and imaging processes can lead to diagnostic errors, and will discuss the role clinical laboratory specialist and radiologists could fulfill in improving the diagnostic process and in reducing diagnostic harm.
First, Paul Epner, a leader in the field of improving the value of laboratory diagnostics for patients, will present his 5-cause framework for errors in the laboratory testing process, focusing on errors in the pre-pre- and post-post-analytical phases of lab testing (i.e. errors in ordering the right test and correct test interpretation).
Next, Prof. Plebani, (clinical biochemist) a laboratory medicine professional with broad experience in measuring errors in the total laboratory testing process, will present data the frequency and nature of these errors and will discuss how use of quality indicators can contribute to decreasing the incidence of diagnostic errors.
Finally, Dr. Cécile Ravesloot, radiologist, will present her research work on improving image interpretation skill by radiologists by introducing authentic images in assessments.
Learning objectives:
- To identify the mechanisms by which laboratory medicine can cause diagnostic errors and strategies for mitigating the risk
- The value of laboratory medicine and how laboratory stewardship will contribute to greater value and to decrease diagnostic errors.
- How radiology expertise can be stimulated by using authentic images for image interpretation in learning and assessment.
Paul Epner
An overview on testing-related error
This session describes the mechanism by which clinical laboratory testing can lead to diagnostic error and then illustrates the leading role laboratory physicians and scientists could take to improve the diagnostic process and reduce diagnostic harm. Opportunities for laboratory professionals to deliver value to patients and the care delivery team through an outcomes-oriented approach will also be described as will effective Interventions and practices that can be implemented to shift from being lab-centric to patient-centered clinical laboratories.
Mario Plebani
Laboratory-Testing Related Error
Over the last decades, more and more timely and accurate laboratory test results have been produced leading the clinical laboratory to be recognized as the “nerve centre of diagnostic process”. Every day, clinical laboratories worldwide analyze billions of samples to provide essential information that allows a reliable clinical decision-making (diagnosis, drugs prescriptions, patients admission/discharge from hospital). However,the complex process that finally provide laboratory information is not error-free. Since 1997, with the publication of the landmark paper by Plebani and Carraro (1), a number of papers demonstrated that errors can occur in any step of the process, in the pre-analytical (46–68.2%), analytical (7–13%) and post-analytical phases (18.5–47%).
Although, compared to billions of laboratory results, the absolute percentage of errors could appear very low, on the contrary, it could become relevant when considered in relation to patient outcome. Although Plebani and Carraro demonstrated that 74% of laboratory errors did not affect patients’ outcome, the other 26% translates into a patient care problem, leading at least to further inappropriate investigations, patient discomfort, increased costs (19%) and, even worse, to inappropriate care and/or modification to therapy (6.4%).
In recent years, a second research path, which has started from the clinical side, demonstrated that errors in laboratory medicine represent a piece of a much wider puzzle that is commonly known as “ diagnostic error ” , definitively linking laboratory-associated errors to patient safety problems.
Recent studies in this area have led to a better understanding of the frequency and nature of diagnostic errors, and their relationship to laboratory testing error. Data on errors in the pre-pre analytical phase (initial procedures performed neither in the clinical laboratory nor, at least in part, under the control of laboratory personnel) underline that failures to order appropriate diagnostic tests, including laboratory tests, accounted for 55% of observed breakdowns in missed and delayed diagnosis in the ambulatory setting and 58% of errors in the Emergency department. In the final steps of the TTP loop, the incorrect interpretation of diagnostic or laboratory tests was found to be responsible for a high percentage of errors in the ambulatory setting as well as in Emergency departments. The search for valuable quality indicators (QIs) for intra- and extra-analytical phases of the testing process and for harmonizing all steps, including test ordering and data interpretation, represents a fundamental issue in projects aiming to improve quality and patient safety.
Cécile Ravesloot
Diagnostic Error in Radiology
Diagnostic radiology errors have gained more attention in the last decades. The prevalence of errors in diagnostic radiology is not completely known, however in the past, rates up to 30 percent were reported (Garland 1949, Berlin 2014). Till know different strategies did not lower these rates much (Berlin 2007). One of these strategies is to learn from what went wrong. All hospitals in the Western world have by now implemented a workflow to safely report errors, scrutinize the process, learn and make changes to the diagnostic process. Increasing performance in image interpretation by improving training and education is another way of reducing errors, which has received less attention. Consequently not much is known about what radiological expertise implies and how it is developed. Therefore radiology training programs and education differ greatly between institutions and did not change much over the years. This in great contrast with the clinical practice of radiologists which is constantly changing.
Radiological image interpretation can be roughly divided in three components: perception, analysis and diagnosis (Van der Gijp 2014). During perception the observer is occupied with detection of abnormalities, and knowledge on normal anatomical structure plays an important role. Analysis implies in depth observation of features of the abnormalities. In the diagnostic phase a conclusion (diagnosis) or action plan for additional diagnostic tests is made. We know from many studies that there is a large inter- and also intra-observer variability in interpreting radiological images (Berlin 2014, Krupinski 2011). So the obvious question is, what makes a radiologist an expert in image interpretation? Image interpretation is traditionally learned in clinical practice, were residents learn from their supervising radiologists. After becoming a registered radiologist in most European countries no formal education and assessment takes places anymore. The common sense was that experience, amount of images read, automatically led to expertise (Manning 2006). However that does not explain the performance differences between experienced radiologists. Furthermore a study in a Dutch cohort of radiology residents showed that mean knowledge growth levels off at average exam scores of 50% with a large dispersion before the end of training (Ravesloot 2017).
In radiology major improvements in imaging and viewing techniques changed needed skills for radiologists completely in the last 15 years. At the end of the 20th century radiologists were viewing printed single slices of cross sectional imaging techniques like Computed Tomography (CT) and Magnetic Resonance Imaging (MRI) hanging next to each (tile viewing). Nowadays all radiological images are digitally viewing and CT and MRI scans, which are often huge volumetric images, can be scrolled through in every possible direction. This is just one of the major changes effecting radiologists’ day-to-day work and different studies showed that the skills for radiological image interpretation changed accordingly. For example, participants interpreting volumetric CT scans were relatively more time occupied with detection of abnormalities in a CT scan than when they were viewing single slices from a CT scan (Van der Gijp 2015). In addition dispersion of scores between high- and low-performers was much larger in assessments with volumetric CT scan than for exams with single slices (Ravesloot 2015a), and in addition volumetric image exams had a better correlation with an external validation measure of volumetric anatomy knowledge (Ravesloot 2015b). Furthermore, in a study of Drew (2013) there appeared to be different strategies of interpretation of volumetric images among radiologists with some evidence that one technique was correlated with better detection rates.
In contrast with the major changes in the viewing task for radiologists, training and education stayed the same for a long time. Students and residents were still trained and assessed with printed single slices most of the time and also no formal training or assessment was given to registered radiologists. Even now new techniques are being implemented in clinical practice without formal training. Also again we are on the brick of major developments with high impact on the radiological imaging process. Artificial intelligence will play a major role in automatic interpretation of images in the near future (Obermeyer 2016). Maybe this will bring us the solution and will cut down error rates finally. However it will never completely take away the interpretation task from humans so we have to keeping improving ourselves and therefore it is essential that education and training keep pace with changing needed skills.
Seminar 4
Art Papier, Jason Maude
Technical solutions
In this session, experts from the field of machine learning will present the current status of machine learning applications for diagnosis and will illustrate this by presenting state-of-the-art solutions.
First, Dr. Art Papier, will talk about machine learning, clinical decision support and diagnosis.
Next, Dr. Kerstin Ritter will present her research work on using computer-based methods (i.e. machine learning) for supporting radiologists in image interpretations.
Finally, Jason Maude will share his thought on different approaches, e.g. rule-based and machine learning, for differential diagnosis generating software.
Learning objectives:
- Understand new strategies in visual representation of diagnosis, machine learning and decision support.
- Learn how computer-based methods can be used to optimize the work of radiologists.
- Pros and cons of the different ways that differential diagnosis generators and symptom checkers are built.
Art Papier
Machine Learning of Skin Presentations
We are the beginning of a revolution in machine learning and artificial intelligence. The visual specialties radiology, dermatology, pathology, and ophthalmology are all seeing striking and rapid advances in machine interpretation of images. In dermatology there has been much focus on machine learning for the detection of skin cancer over the past decade. More recently progress has been made in general diagnosis of skin clues by machine learning. In this session we will discuss how machine learning can be integrated into broad diagnostic support, and how visual representation of medical diagnostic complexity enhances both System 1 and System 2 cognition.
Jason Maude
Deep Learning versus Rules Based DDx Generators
With the proliferation of DDx Generators and Symptom Checkers it’s important for clinicians to understand the big differences in how they are built as this determines their capabilities. In this talk we will look at the key methods of construction, some examples and suggest criteria clinicians can use for evaluating them.
Workshops 5
Paul Epner
Using Quality Improvement Techniques to reduce Diagnostic Error
Health care systems are continually involved in quality improvement, but rarely directed at diagnostic error. We are early in the development of effective interventions. In this workshop, we will share case studies of what works, hear the evolution of a collaborative that sought to test some of these interventions, and in a highly interactive session, determine strategies for implementing diagnostic error quality improvement in your clinical setting now.
Seminar 6
David Newman-Toker
Stroke Misdiagnosis
Worldwide, there are more than 15 million strokes annually, roughly one third of which result in death. In the US, stroke is the most commonly missed vascular event and results in both the most malpractice claims and the most total payouts. Strokes are most often missed when symptoms are transient, mild, or non-specific. The symptom most tightly linked to missed stroke in frontline healthcare settings is dizziness/vertigo. Causes appear disproportionately related to misinformation and lack of expertise in bedside assessment. Novel approaches to teamwork, training, technology, and tuning may facilitate timely, accurate diagnosis, thereby preventing serious misdiagnosis-related harms, including permanent disability and death.
Learning Objectives:
- Describe the clinical contexts in which strokes are most frequently missed and the overall burden of harms.
- List the principal causes of stroke misdiagnosis, comparing cognitive and systems contributions.
- Discuss novel solutions to improve stroke diagnosis, leveraging technology and telemedicine.
Yoryos Lyratzopoulos
Improving the Diagnosis of Cancer: Recent UK Initiatives, Ongoing Research Priorities, Potential Lessons for other Disease Areas and Health Systems
Since 2008, the UK government and major charitable research funders have supported a range of policy initiatives, together with population health and healthcare system research projects, to improve the diagnosis of cancer. These policies, which were prompted by prior epidemiological research documenting unfavourable international comparisons for cancer outcomes in English patients, have since transformed both the evidence base and the configuration of health services and pathways for diagnosing cancer in symptomatic patients. Professor Lyratzopoulos will highlight key achievements in this transformational journey and summarise recent research findings. He will draw out the potential usefulness of UK research and policy to improve the diagnosis of cancer for other disease areas and health systems.
Juliane Kämmer, Wolf Hautz
Diagnostic Error in Emergency Medicine
Many diagnoses are first made in the emergency department, which is particularly prone to error: information is limited and sometimes unreliable, time pressure and workload are high and diagnosticians work in ad hoc teams with interfaces to many colleagues from ever changing disciplines. What is more is that emergency diagnoses (and errors therein) are often particularly consequential for patients and diagnosticians alike.
In this talk, the authors will present their findings from a variety of studies. They will report on the epidemiology and clinical consequences of diagnostic error in the emergency room, provide an overview of their work regarding collaborative diagnoses in ill-defined decision environments and briefly touch on the difficulty of simply adopting solutions from other high-risk industries such as aviation.
Latest Research in Improving Diagnosis
Looking for more research? Check out the Society to Improve Diagnosis in Error (SIDM)'s peer-reviewed journal Diagnosis for the latest developments in improving diagnosis.
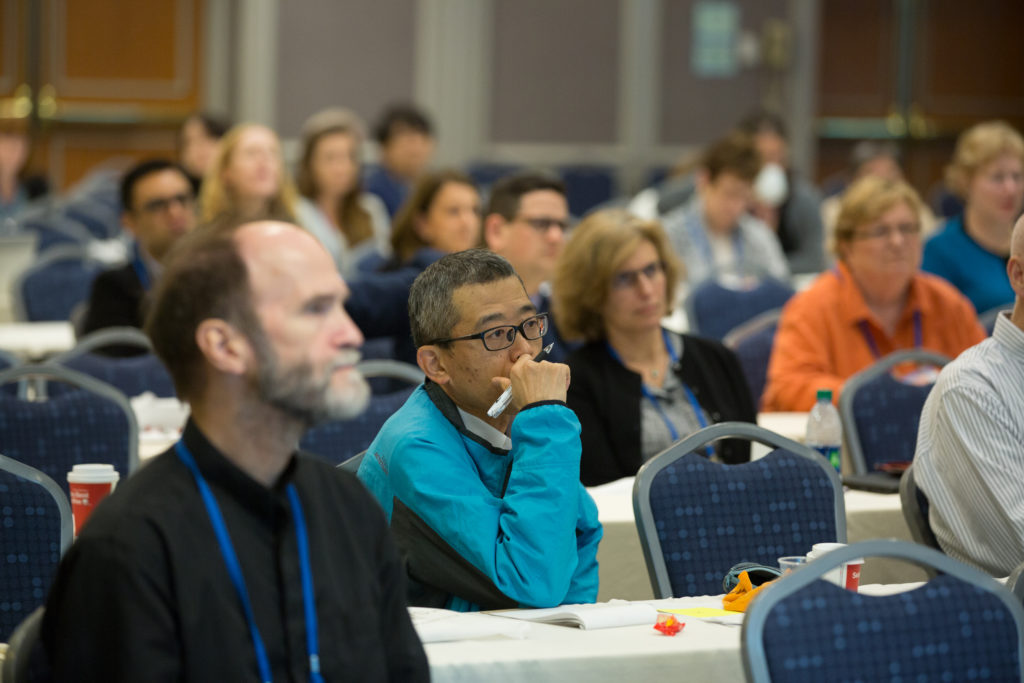
Support Our Mission
SIDM catalyzes and leads change to improve diagnosis and eliminate harm from diagnostic error, in partnership with patients, their families, the healthcare community and every interested stakeholder.